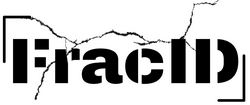 |
Parameter estimation framework for fracture propagation problems under extreme mechanical loads
|
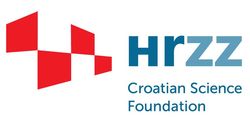 |
This project was funded by the Croatian Science Foundation under the grant UIP-2017-05-3429.
|
Project Summary
Correct prediction and prevention of catastrophic failure events in engineering structures and materials represent complex engineering and scientific challenge. Even when using sophisticated nonlinear numerical models that can simulate failure mechanisms induced by fracture initiation and propagation, outputs cannot be considered reliable if the material parameters are uncertain. The project proposal aims to develop a methodology for the reliable estimation of fracture parameters. The methodology will be based on a solution of stochastic inverse problem that combines measurements and a computational model. In this project we will use so-called synthetic measurements that serve for the methodology development, while our research group will develop the framework based on the probability theory with Bayesian inference and novel numerical fracture model. Fracture parameters depend on unknown microstructural composition of the material with its defects and imperfections, which makes them uncertain. Initial knowledge about uncertain parameters will be represented with prior probability distributions that will be updated to posterior distributions by using measurements, probability theory and the fracture model. The novel lattice numerical fracture model will be developed based on our previous research and embedded strong discontinuities for localized failure with the novelty related to computation of lattice elastic parameters. Monte Carlo Markov Chain and Kalman filter methods will be implemented and used in estimating fracture parameters of steel and concrete subjected to extreme loads. The methodology will also be able to quantify uncertainties that arise from microstructure resulting with deeper understanding of the physical problem and better decision making strategies. Reliable estimation of fracture parameters will enhance our predictive modelling strategies either when designing new structures or estimating the integrity and carrying capacity of existing.
Project Team
- Assist. prof. Mijo Nikolić, PhD (principal investigator)
- Assist. prof. Andjelka Stanić, PhD (researcher)
- Assist. prof. Eduard Marenić, PhD (researcher)
- Matej Šodan, MCEng (PhD student)
- Nives Brajčić Kurbaša, PhD (researcher)
Assist. prof. Mijo Nikolić, PhD, is a principal investigator (PI). His research is related to numerical modelling, fracture propagation problems, multi-physics problems related to porous medium and soil mechanics. His main role is to work on the development of the new simulation tools related to fracture, as well as development of stochastic toolbox that will be used for reliable estimation of fracture parameters and more accurate prediction tools for fracture propagation problems.
Assist. prof. Andjelka Stanić, PhD, is a member of the research team. She is employed at University of Twente, Netherlands. Her research topic is related to fracture propagation problems and stochastic analysis.
Assist. prof. Eduard Marenić, PhD, is a member of the research team. He is employed at Institut Clement Ader (ICA), Université de Toulouse, France. His research topic is related to data-driven mechanics.
Matej Šodan, MCEng, is a PhD student working on the project objectives related to development of fracture models and stochastic identification of parameters.
Nives Brajčić Kurbaša, PhD, is a researcher working on project objectives. She is employed at the Faculty of Civil Engineering, Architecture and Geodesy, University of Split.
Project Goals
- Development of methodology for solution of stochastic inverse problem
- Development of novel fracture model
- Steel parameter estimation in impact dynamics test
- Concrete parameter estimation
- Collection of project results, methodology experiences and engineering applications
- Supervision of a PhD thesis